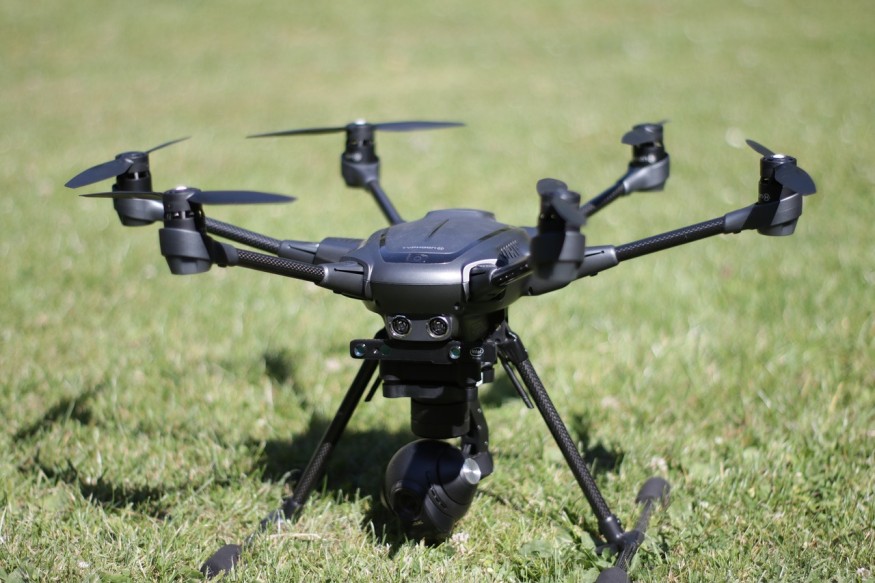
With the use of machine or AI learning, researchers are able to analyze images from drones taking shots over the soil, and understand the responses of root crops to heat and drought below the soil. Scientists use the Pheno-i platform to relay data in real-time and be able to help breed crops that are more resilient to climate change.
It is very hard to detect nutritional deficiencies and diseases in many root crops such as carrots, potatoes, and cassava. These problems affect their ability to propagate and grow. Even with illnesses and deficiencies in nutrition, they are able to look healthy, with green leaves that look robust and problem-free. It is only when these crops are harvested that farmers get the shocking revelation that they have poorly grown farm produce.
It is also a problem for many plant breeders because they need to wait for many months and even years before they are able to see if their crops have negatively responded to weather problems such as temperature changes and drought. The productivity of crops is also affected when the farmer does not know the required growing conditions and nutrients of the crop in the planting and early growth stages.
The new study makes use of machine learning in order to predict the health and growth of roots using above-ground images. It was recently published on June 14 in the journal Plant Methods.
Study co-author Michael Selvaraj from Alliance of Bioversity International and CIAT says that whether what is seen to be occurring above the ground also occurs below the ground is considered a great mystery by plant breeders. Crop physiologist Selvaraj says that it is also a conundrum for researchers and scientists. They must conduct many trials and gather many types of information, such as plant height, canopy, and many other plant features before they can really assess if it is actually healthy or if it just appears to be healthy. This information gathering and trial process is very time-consuming and takes extensive energy.
Even if the cost of drones is progressively becoming lower and the method for gathering visual images in crops is now much easier, it is still very difficult to sort and analyze the enormous volume of visual data. Furthermore, these must be distilled in order to become useful for breeders and farmers.
With the drone images provided by Pheno-I, data from thousands of high-res pictures can now be merged and analyzed with the use of machine learning. The end product is a spreadsheet that shows the exact conditions of the crops in the field, and if they are responsive to stimuli. The results provided are also produced in real-time.
With this technology to help them, breeders will be able to immediately respond to threats by quickly providing the lacking nutrients, give more water if needed, or treat the plants if necessary.
The machine-produced data also lets researchers quickly detect which of the crops can better resist climate changes and shocks, helping crop farmers choose more heat-resilient and drought-resistant crop varieties.
Selvaraj says that they are helping breeders more quickly choose the best varieties that are higher-yielding and better resistant to climate changes. He says the analytics platform helps them provide actionable and useful data that can accelerate the productivity of crops.
The Alliance's Crops for Nutrition and Health research director Joseph Tohme says that this platform can also be used for other crops.
© 2025 NatureWorldNews.com All rights reserved. Do not reproduce without permission.